Artificial intelligence (AI) and machine learning (ML) are often used interchangeably, but they are not the same thing.
AI is a broader concept that refers to the ability of machines to perform tasks that normally require human intelligence, such as reasoning, decision-making, and natural language processing.
ML is a subset of AI that enables machines to learn from data and improve their performance without being explicitly programmed.
But can AI exist without ML?
Is there a way to create intelligent machines without relying on data-driven algorithms?
And what are the advantages and disadvantages of doing so?
In this article, we will explore these questions and provide some examples of AI without ML.
We will also discuss why it is important to understand the difference between AI and ML, and how they can complement each other in various applications.
What is AI Without ML?
AI without ML is a type of AI that does not use data or statistical methods to learn from experience but rather relies on predefined rules, logic, or knowledge bases. This type of AI is sometimes called symbolic AI, rule-based AI, or good old-fashioned AI (GOFAI).
AI without ML was the dominant approach to AI in the early days of the field, before the advent of ML in the late 1970s and 1980s.
Researchers and engineers tried to encode human knowledge and reasoning into symbolic systems that could manipulate symbols according to formal rules.
For example, expert systems were developed to emulate the decision-making of human experts in domains such as medicine, law, or engineering.
These systems used a set of if-then rules or facts stored in a knowledge base to infer conclusions or recommendations based on user input.
Another example of AI without ML is natural language processing (NLP), which involves understanding and generating natural language.
Early NLP systems used grammar, dictionaries, and parsers to analyze and produce sentences based on syntactic and semantic rules.
For instance, chatbots were designed to simulate human conversation by matching user input to predefined responses or templates.
What Are the Pros and Cons of AI Without ML?
AI without ML has some advantages and disadvantages compared to AI with ML. Here are some of them:
Pros of AI Without ML
Transparent and Explainable
AI without ML can be more transparent and explainable than AI with ML.
Since the logic and rules behind the system are explicitly defined and human-readable, it is easier to understand how and why the system makes certain decisions or outputs.
This can increase trust and accountability in the system, especially in sensitive or critical domains such as healthcare, finance, or law.
Robust and Reliable
AI without ML can be more robust and reliable than AI with ML. Since the system does not depend on data quality or quantity, it is less prone to errors, biases, or overfitting.
The system can also handle edge cases or exceptions that may not be covered by data.
Moreover, the system can be more consistent and predictable in its behavior, as it does not change or adapt over time unless explicitly modified.
Efficient and Scalable
AI without ML can be more efficient and scalable than AI with ML.
Since the system does not require data collection, preprocessing, storage, or analysis, it can save time, resources, and costs.
The system can also run faster and use less computational power than data-intensive algorithms.
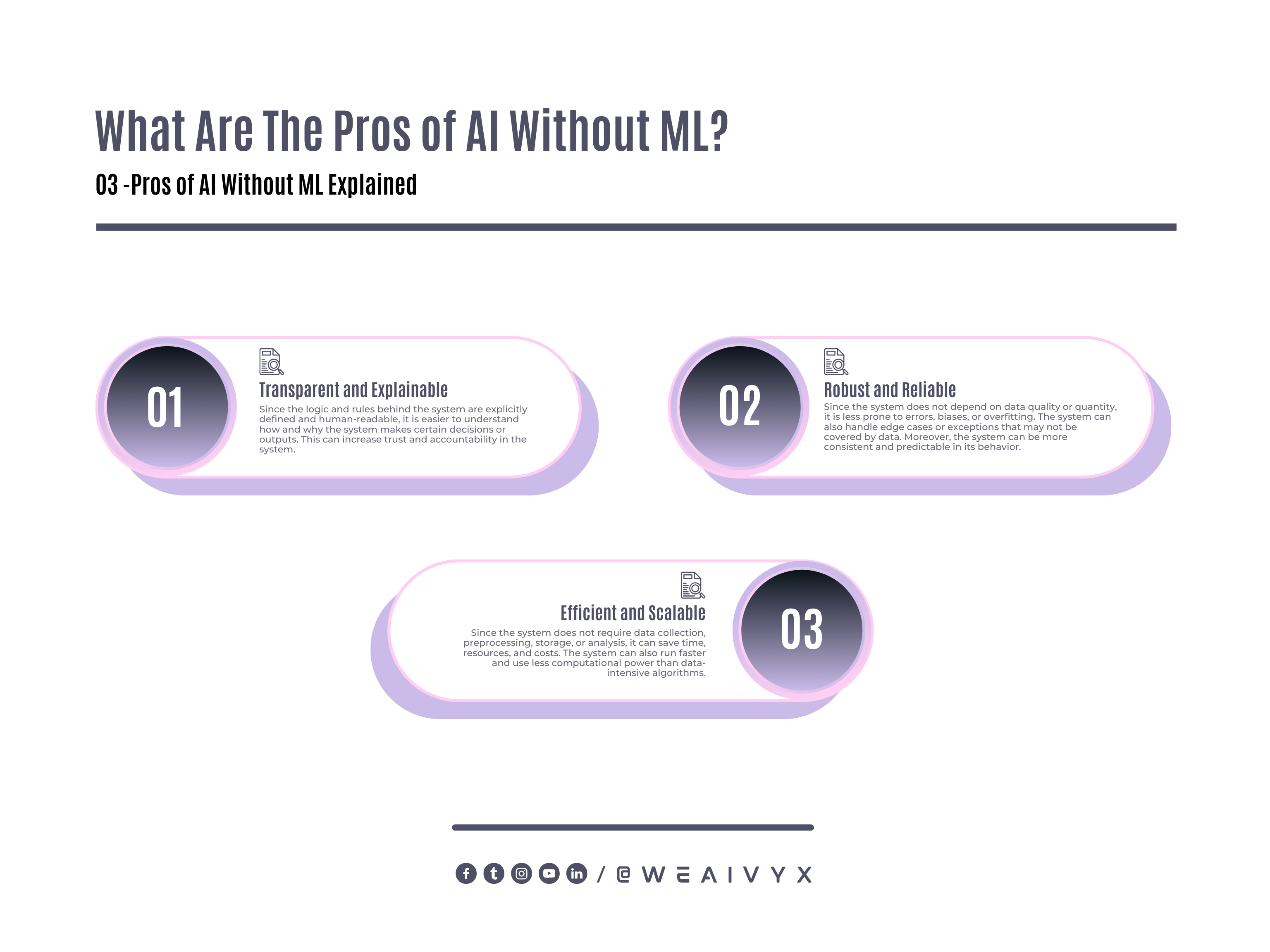
Cons of AI Without ML
Limited and Rigid
AI without ML can be more limited and rigid than AI with ML. Since the system relies on predefined rules or knowledge, it cannot handle situations that are novel, complex, or ambiguous.
The system may also lack creativity or flexibility in generating solutions or responses that are not already programmed.
Furthermore, the system may become outdated or obsolete as new information or knowledge emerges.
Labor-Intensive and Costly
AI without ML can be more labor-intensive and costly than AI with ML.
Since the system requires manual input from experts or programmers, it can be time-consuming and challenging to design, develop, maintain, and update.
The system may also suffer from human errors or biases that are hard to detect or correct.
Less Accurate and Effective
AI without ML can be less accurate and effective than AI with ML.
Since the system does not learn from data or feedback, it cannot improve its performance or optimize its outcomes.
The system may also miss important patterns or insights that are hidden in data.
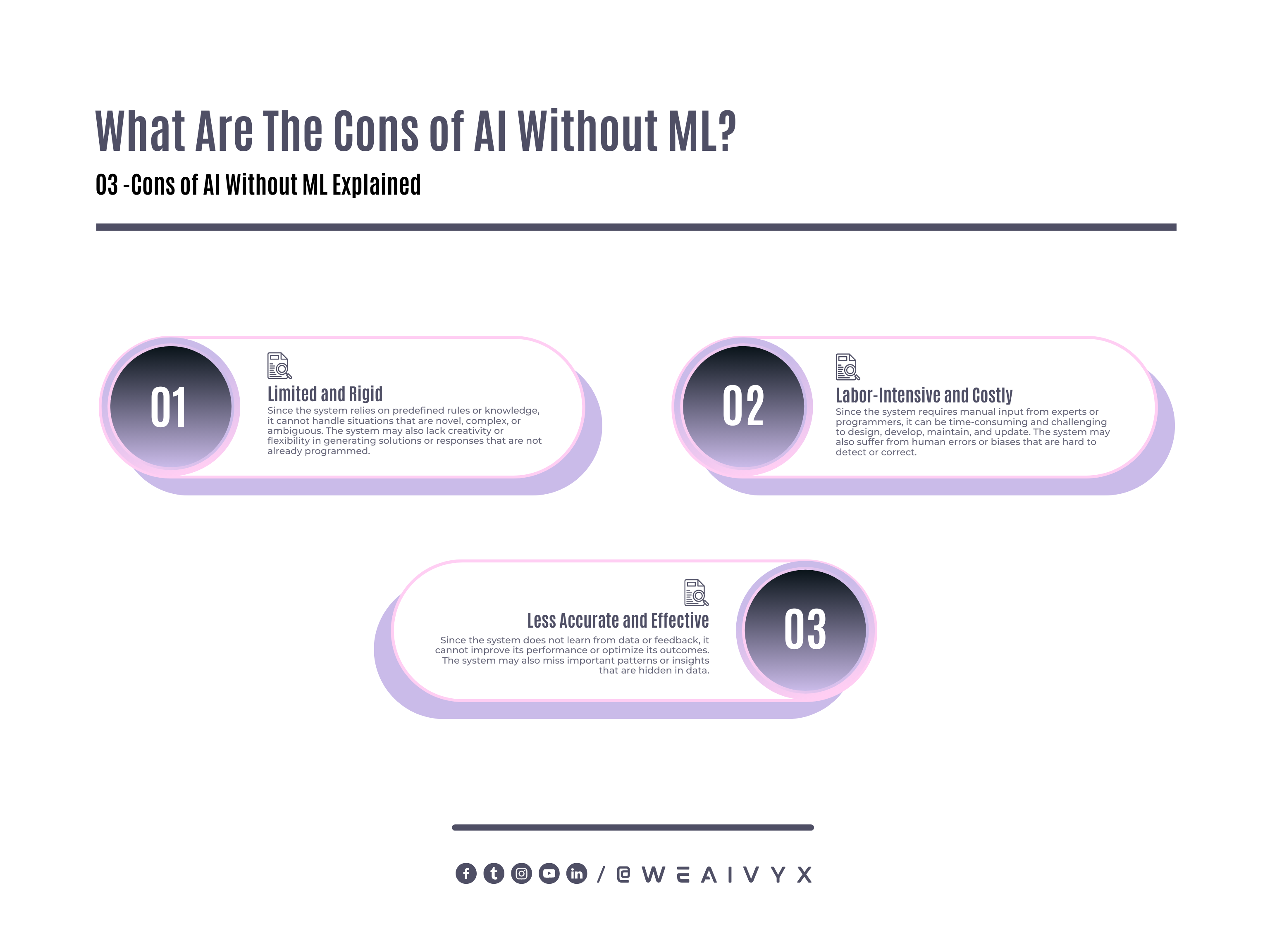
How Can AI With ML and AI Without ML Work Together?
One way is to combine symbolic reasoning with data-driven learning in a hybrid system that leverages the strengths of both methods.
For example, knowledge graphs are a type of symbolic representation that stores entities and relations in a graph structure.
By applying ML techniques such as graph neural networks or embeddings on knowledge graphs, one can enhance their expressiveness and scalability while preserving their interpretability and logic.
Another way is to use symbolic systems as prior knowledge or a constraint or a guide for data-driven learning in a complementary system that reduces the search space or the complexity of the problem.
For example, neural symbolic learning is a paradigm that integrates symbolic representations and neural networks to enable both deductive and inductive reasoning.
By using symbolic rules or knowledge as a prior, one can improve the generalization and explainability of neural networks while retaining their power and flexibility.
A third way is to use data-driven systems as a source of data or feedback for symbolic systems in a collaborative system that enhances the learning and adaptation of both methods.
For example, reinforcement learning is a type of ML that enables agents to learn from their actions and rewards in an environment.
By using symbolic systems as a model of the environment or the agent’s goals, one can improve the efficiency and effectiveness of reinforcement learning while incorporating domain knowledge and logic.

Why Does It Matter to Understand the Difference Between AI and ML?
Understanding the difference between AI and ML is not only important for technical reasons, but also for ethical, social, and economic reasons. Here are some of them:
Avoid Confusion and Misinformation
Understanding the difference between AI and ML can help us avoid confusion and misinformation about the capabilities and limitations of AI systems.
For example, some people may have unrealistic expectations or fears about AI based on popular media or hype, without realizing that AI is not a monolithic or homogeneous phenomenon, but rather a diverse and heterogeneous field with different subfields, methods, applications, and challenges.
Make Informed and Responsible Decisions
Understanding the difference between AI and ML can help us make informed and responsible decisions about the design, development, deployment, and governance of AI systems.
For example, some AI systems may require more human oversight or intervention than others, depending on their level of autonomy, adaptability, and accountability.
Some AI systems may also pose more risks or benefits than others, depending on their impact on human rights, values, and interests.
Oster Innovation and Collaboration
Understanding the difference between AI and ML can help us foster innovation and collaboration in the AI community and beyond.
For example, some AI problems may require interdisciplinary or cross-disciplinary approaches that combine different types of AI or other fields such as psychology, sociology, philosophy, or law.
Some AI solutions may also benefit from open-source or crowdsourced initiatives that involve diverse stakeholders such as researchers, developers, users, regulators, or civil society.
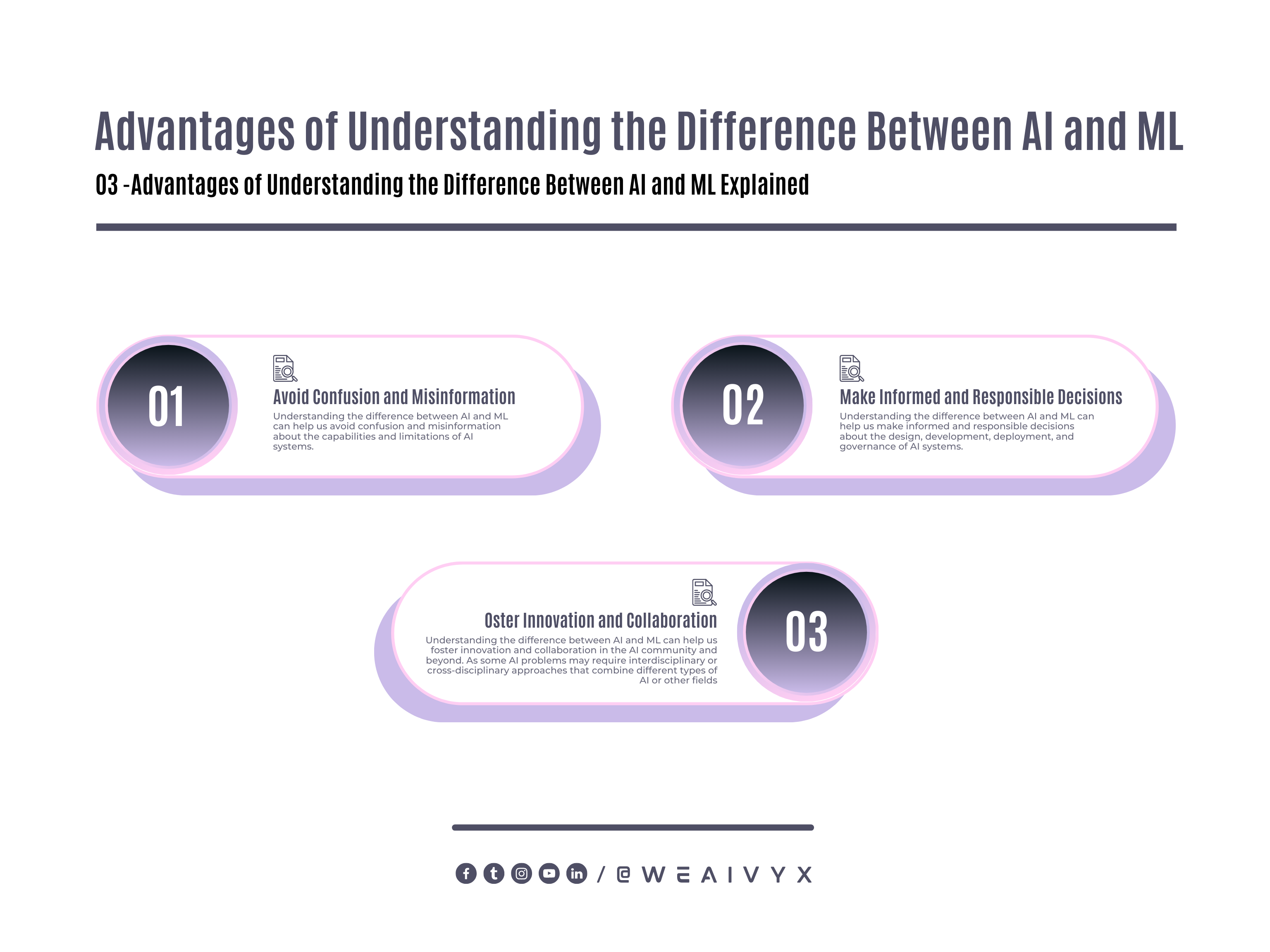
Conclusion
AI without ML is a type of AI that does not use data or statistical methods to learn from experience but rather relies on predefined rules, logic, or knowledge bases.
AI without ML has some advantages and disadvantages compared to AI with ML, such as transparency, robustness, efficiency, scalability, limitation, rigidity, labor intensity, cost, accuracy, and effectiveness.
AI with ML and AI without ML are not mutually exclusive approaches to creating intelligent machines.
They can work together in various ways to achieve better results than either approach alone, such as combining symbolic reasoning with data-driven learning, using symbolic systems as a piece of prior knowledge or a constraint for data-driven learning, or using data-driven systems as a source of data or feedback for symbolic systems.
AI without ML is not obsolete or irrelevant in the era of big data and deep learning. Rather, it is an important and valuable aspect of AI that can complement and enhance the capabilities of data-driven systems.
By understanding the difference between AI and ML, and how they can work together, we can create more powerful, reliable, and responsible AI systems for various applications.
If you enjoyed this article, please share it with your friends or colleagues who are interested in AI. If you have any questions or comments, please leave them below.